Experiments - Part 2
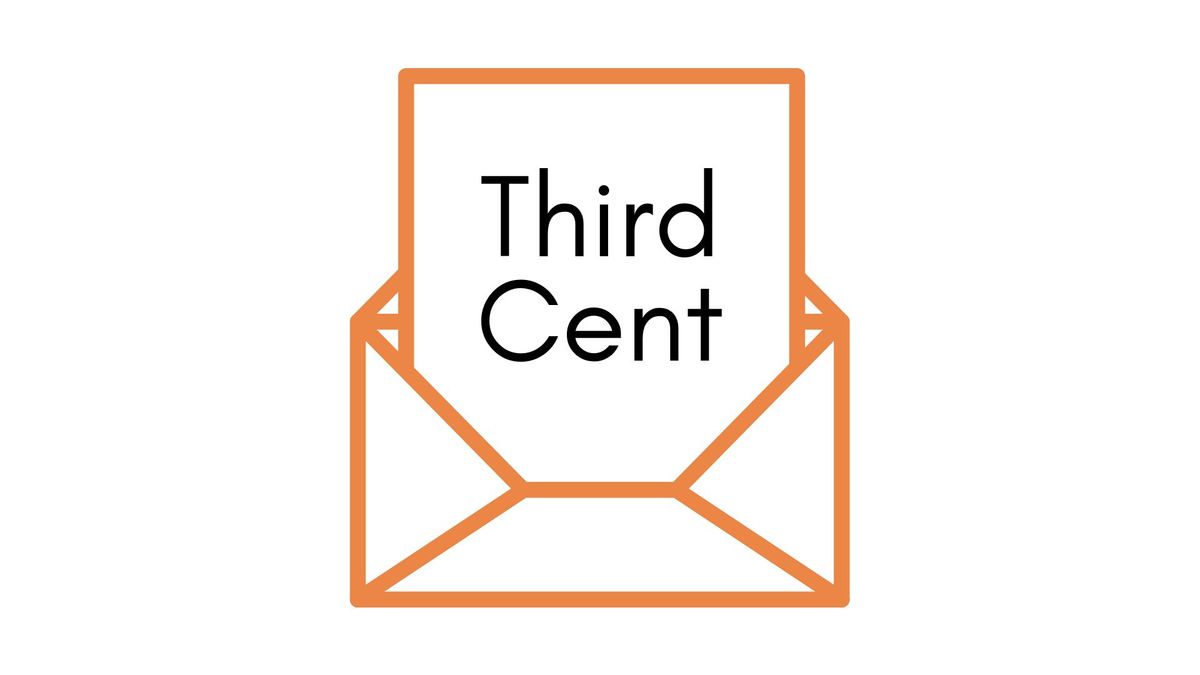
Introduction
Remember the YouTube Short from the previous newsletter. Just in case you forgot, here’s the link. Please do watch it again.
Now that you have watched it, let’s perform a little thought experiment.
If we were to prove dinitrophenol (DNP) had deadly effects (including but not limited to weight loss), how would we go about doing that using an RCT?
The simplest way to go about it is to get two completely random samples of people. Give one of the samples DNP and the other some placebo. Advice all participants to maintain a certain type of eating habits and observe the two for a few months or years. If DNP had a detrimental effect on health, then the sample that was given DNP would experience severe weight loss and face other difficulties. If not, both the samples (control and treatment groups) would have similar outcomes. However, as a scientist, knowing fully well that DNP could cause severe health issues, would you be able to conduct an RCT? Would it be ethical to subject unknowing individuals to this? (If you are interested in this story, learn more about it here)
The answer is, of course, No! It is true that randomized control trials (RCTs) are the gold standard of causal inference. It is also true that they can identify the existence of treatment effects and are able to estimate them quite accurately. However, it is not always possible for scholars to use RCTs.
They can be unethical, costly, or impractical to execute in many instances. This is where natural experiments or quasi experimental methods come into the picture. In brief, quasi experimental methods are research designs that that aim to “identify the impact of a particular intervention, program, or event (a "treatment") by comparing treated units (households, groups, villages, schools, firms, etc.) to control units.” (Source). They are increasingly being used in business research contexts as well. While there are many natural experiment methods, we shall limit our discussion to two specific methods – (1) the difference in differences, and (2) the regression discontinuity approach.
Difference in Differences:
Simply put, the difference in differences (DID) is a causal inference technique that is used to estimate the impact of a policy change on a treated group and a control group. Unlike in the case of the RCT where we ensure randomization, here we assume that the control and treatment groups tend to move in similar ways (this is more commonly referred to the parallel trends assumption). Therefore, the difference in the post-treatment outcome for the treated group and the post-treatment outcome for the control group minus the difference in the pre-treatment outcome for the treated group and the pre-treatment outcome for the control group gives us an estimate of the casual effect. Was that difficult to process in text form? Let me illustrate to make things clearer. To illustrate, let me use a table from UICEF’s note on natural experiments. (you can find the same here )
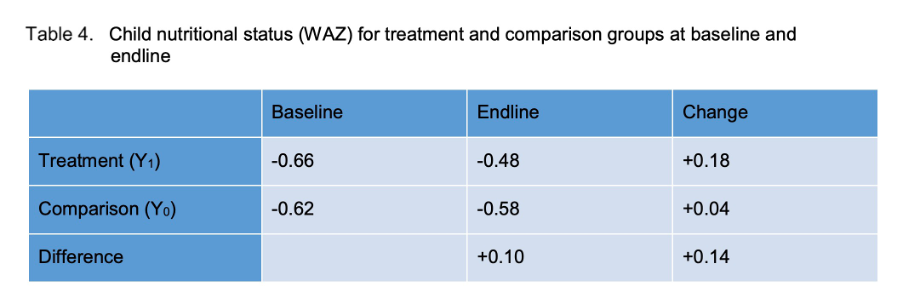
Here, the treatment and control (referred to as comparison) group have similar baseline scores (measure in terms of weight for age z scores – a commonly used health measure). However, in the treatment group, a nutritional supplementation programme was carried out. Nothing was done in the control/comparison group. Therefore, we should ideally see some change in the treatment group. As expected, you can see that there is a +0.18 change. However, you cannot really call this the treatment effect. This is a composite of two effects, one of which is the treatment. The other is the temporal effect that both the groups feel regardless. That is, assuming the treatment and control groups behave similarly, the treatment group too should have experienced a change of +0.04 in weight for age z scores. So, we must subtract this time trend from the total change. In effect, we find the difference in difference.
If this sounds too complicated, please do not worry. Just know that there is a way to measure the effects of certain treatments even if you do not meticulously plan an RCT. There is however, some issues about which we should exercise care when using the DID. The DID assumes that there is a parallel trend between the two groups, the two groups are equivalent, and that the treatment is carried out randomly. This may not be the case. Often, interventions are carried out only in locations where they are likely to succeed. This issue can be handled to some extent by using matched difference in differences. In matched difference in differences, the two groups are ‘matched’ based on various characteristics that matter. You should get Googling if you’d like to learn more about this technique. Such assumptions are not made in the next technique that we shall explore- the regression discontinuity designs.
Regression Discontinuity Designs
Regression discontinuity design (RDD) is a type of quasi-experimental research design that is used to evaluate the effect of a treatment or intervention on an outcome variable. It involves comparing the outcomes of individuals who are just above and just below a certain threshold for receiving the treatment or intervention.
In an RDD study, the threshold for receiving the treatment is chosen in such a way that individuals just above and just below the threshold are similar in all other characteristics except for their exposure to the treatment. This allows researchers to isolate the effect of the treatment and control for other factors that could influence the outcome.
For instance, let’s consider our attendance data (pictorial representation found below). Recall that the date when I mandated the email approval requirement was arbitrarily decided, it stands to reason that the days before and the days after are very similar in nature. Everything was the same except the fact that the new rule was implemented.
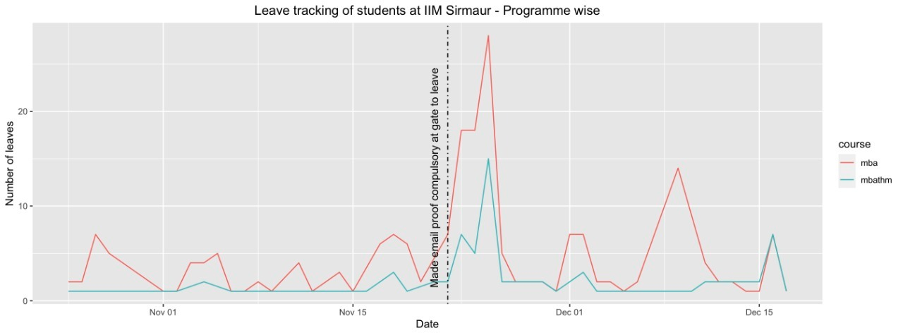
Therefore, the difference in the outcome (number of leaves taken) before and after the dates is on account of the new rule getting implemented. In many ways, the RDD can provide more accurate estimates of the treatment effect compared to other research designs (such as DID) because it controls for the possibility that individuals who receive the treatment differ from those who do not in ways that could affect the outcome.
If you want to explore more about the RDD, please do check out the following links:
1. https://www.princeton.edu/~davidlee/wp/RDDEconomics.pdf
They offer a comprehensive overview of the method and lay the groundwork for further exploration.
All the best, see you in class tomorrow!